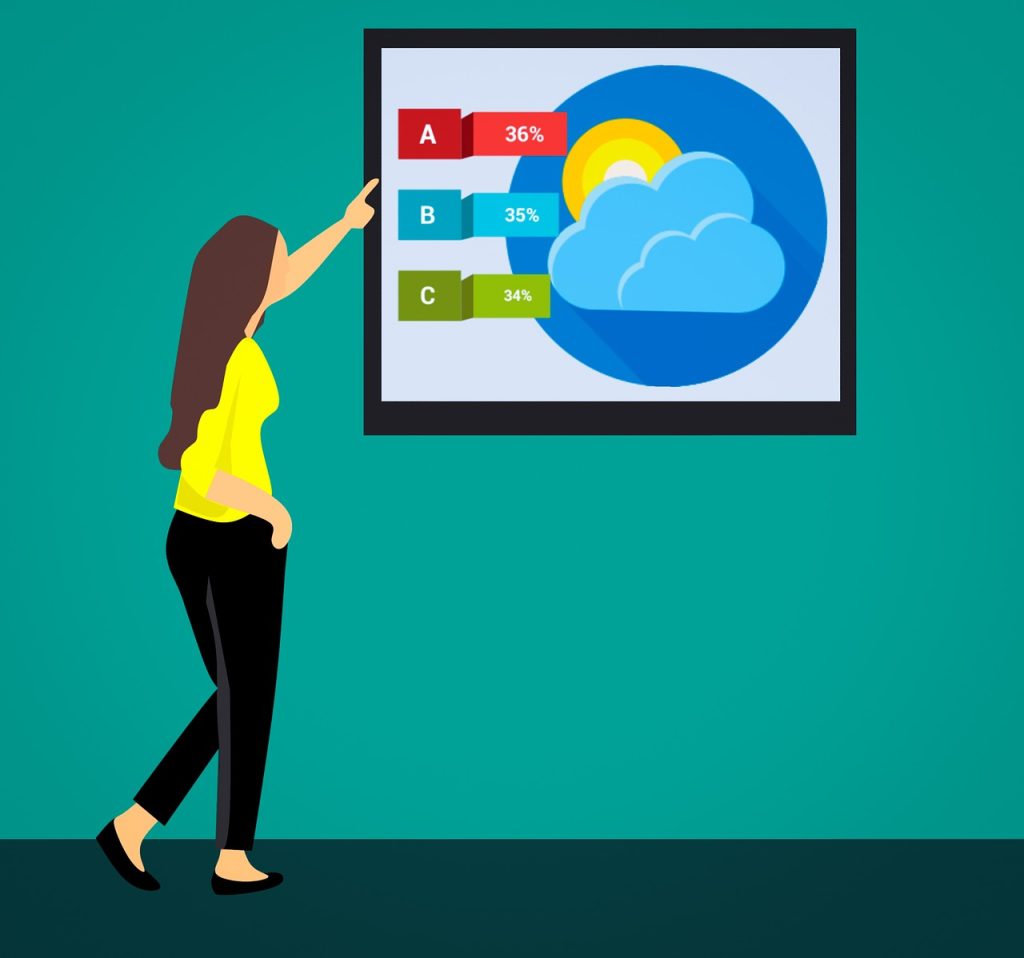
Have you ever wondered how Netflix knows what show you’ll love to watch next or how Amazon recommends products that seem to be made just for you? The answer is predictive analytics, a powerful tool that allows businesses to make accurate predictions about future events or trends.
In fact, predictive analytics is not something that is particularly new. All sorts of websites and digital services have been using predictive analytics for some time already. A personal favourite of ours is the application of content recommendations to predict what we want to read next online.
Implementing predictive analytics begins with being able to analyse historical data first. At its most basic, you could take a user’s usual behaviour to read news about Manchester United football club and serve up the latest news about them all the time. A bit more lateral thinking might lead you to the conclusion to test serving news about local rivals Manchester City instead. What’s to say that fans don’t want to read about the travails of their nearest competitor?
Predictive analytics is almost everywhere now in modern day digital marketing. We see it in content recommendation tools, we see it in advertising networks like Google and Facebook. It’s based on being able to analyse historical data to spot where there might be patterns. Those patterns can be used to make predictions. For a marketer, you can think about what it means to serve an ad to a particular user or not. That is effectively predictive analytics, to predict if a user will click a certain creative or not.
However, it’s worth noting that there are no guarantees with predictive analytics. It’s highly unlikely that you’ll see any model that has 100% accuracy. The reality is that there will always be some errors. The challenge is to minimise the errors as much as possible. The quality and accuracy of the model will always be dependent on the data that is used to train it.
Let’s pause for a moment and consider a grey area related to predictive analytics – ethics. Just because you can do something, doesn’t mean that you should. There will be question marks about what data is getting processed, and practitioners of machine learning must be careful with whether or not personal identifiable information is used in the process. Privacy should never be sacrificed for the sake of predictive analytics deployment.
For a digital marketer, what could you do with predictive analytics? Well, we’ve already mentioned how ad networks are natively using it in optimisation, but you can also use it with something such as forecasting. With the right type of technical skills, it’s possible for you to carry out some simple forecasting of data. You might even use some simple machine learning to try to predict how effective subject lines or ad copy would be. These are just some very specific examples of predictive analytics, but rest assured that the world is big, and marketing has much more potential for further applications of predictive analytics.